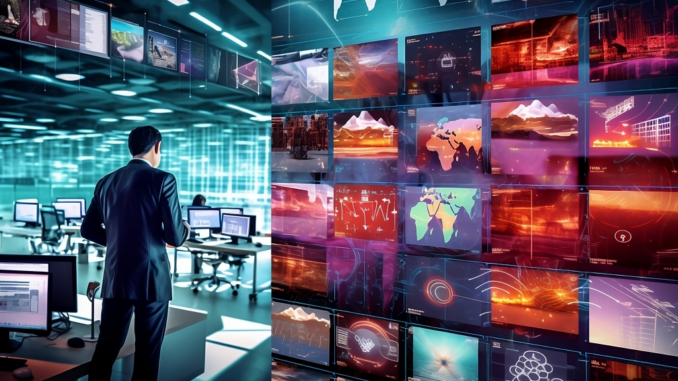
Join our daily and weekly newsletters for the latest updates and exclusive content on industry-leading AI coverage. Learn More
Intelligence is pervasive, yet its measurement seems subjective. At best, we approximate its measure through tests and benchmarks. Think of college entrance exams: Every year, countless students sign up, memorize test-prep tricks and sometimes walk away with perfect scores. Does a single number, say a 100%, mean those who got it share the same intelligence — or that they’ve somehow maxed out their intelligence? Of course not. Benchmarks are approximations, not exact measurements of someone’s — or something’s — true capabilities.
The generative AI community has long relied on benchmarks like MMLU (Massive Multitask Language Understanding) to evaluate model capabilities through multiple-choice questions across academic disciplines. This format enables straightforward comparisons, but fails to truly capture intelligent capabilities.
Both Claude 3.5 Sonnet and GPT-4.5, for instance, achieve similar scores on this benchmark. On paper, this suggests equivalent capabilities. Yet people who work with these models know that there are substantial differences in their real-world performance.
What does it mean to measure ‘intelligence’ in AI?
On the heels of the new ARC-AGI benchmark release — a test designed to push models toward general reasoning and creative problem-solving — there’s renewed debate around what it means to measure “intelligence” in AI. While not everyone has tested the ARC-AGI benchmark yet, the industry welcomes this and other efforts to evolve testing frameworks. Every benchmark has its merit, and ARC-AGI is a promising step in that broader conversation.
Another notable recent development in AI evaluation is ‘Humanity’s Last Exam,’ a comprehensive benchmark containing 3,000 peer-reviewed, multi-step questions across various disciplines. While this test represents an ambitious attempt to challenge AI systems at expert-level reasoning, early results show rapid progress — with OpenAI reportedly achieving a 26.6% score within a month of its release. However, like other traditional benchmarks, it primarily evaluates knowledge and reasoning in isolation, without testing the practical, tool-using capabilities that are increasingly crucial for real-world AI applications.
In one example, multiple state-of-the-art models fail to correctly count the number of “r”s in the word strawberry. In another, they incorrectly identify 3.8 as being smaller than 3.1111. These kinds of failures — on tasks that even a young child or basic calculator could solve — expose a mismatch between benchmark-driven progress and real-world robustness, reminding us that intelligence is not just about passing exams, but about reliably navigating everyday logic.
The new standard for measuring AI capability
As models have advanced, these traditional benchmarks have shown their limitations — GPT-4 with tools achieves only about 15% on more complex, real-world tasks in the GAIA benchmark, despite impressive scores on multiple-choice tests.
This disconnect between benchmark performance and practical capability has become increasingly problematic as AI systems move from research environments into business applications. Traditional benchmarks test knowledge recall but miss crucial aspects of intelligence: The ability to gather information, execute code, analyze data and synthesize solutions across multiple domains.
GAIA is the needed shift in AI evaluation methodology. Created through collaboration between Meta-FAIR, Meta-GenAI, HuggingFace and AutoGPT teams, the benchmark includes 466 carefully crafted questions across three difficulty levels. These questions test web browsing, multi-modal understanding, code execution, file handling and complex reasoning — capabilities essential for real-world AI applications.
Level 1 questions require approximately 5 steps and one tool for humans to solve. Level 2 questions demand 5 to 10 steps and multiple tools, while Level 3 questions can require up to 50 discrete steps and any number of tools. This structure mirrors the actual complexity of business problems, where solutions rarely come from a single action or tool.
By prioritizing flexibility over complexity, an AI model reached 75% accuracy on GAIA — outperforming industry giants Microsoft’s Magnetic-1 (38%) and Google’s Langfun Agent (49%). Their success stems from using a combination of specialized models for audio-visual understanding and reasoning, with Anthropic’s Sonnet 3.5 as the primary model.
This evolution in AI evaluation reflects a broader shift in the industry: We’re moving from standalone SaaS applications to AI agents that can orchestrate multiple tools and workflows. As businesses increasingly rely on AI systems to handle complex, multi-step tasks, benchmarks like GAIA provide a more meaningful measure of capability than traditional multiple-choice tests.
The future of AI evaluation lies not in isolated knowledge tests but in comprehensive assessments of problem-solving ability. GAIA sets a new standard for measuring AI capability — one that better reflects the challenges and opportunities of real-world AI deployment.
Sri Ambati is the founder and CEO of H2O.ai.
Be the first to comment